Future of Education: Multimodal AI?
How Multimodal AI systems are Poised to Revolutionise Education.
Harnessing the Power of Multimodal AI
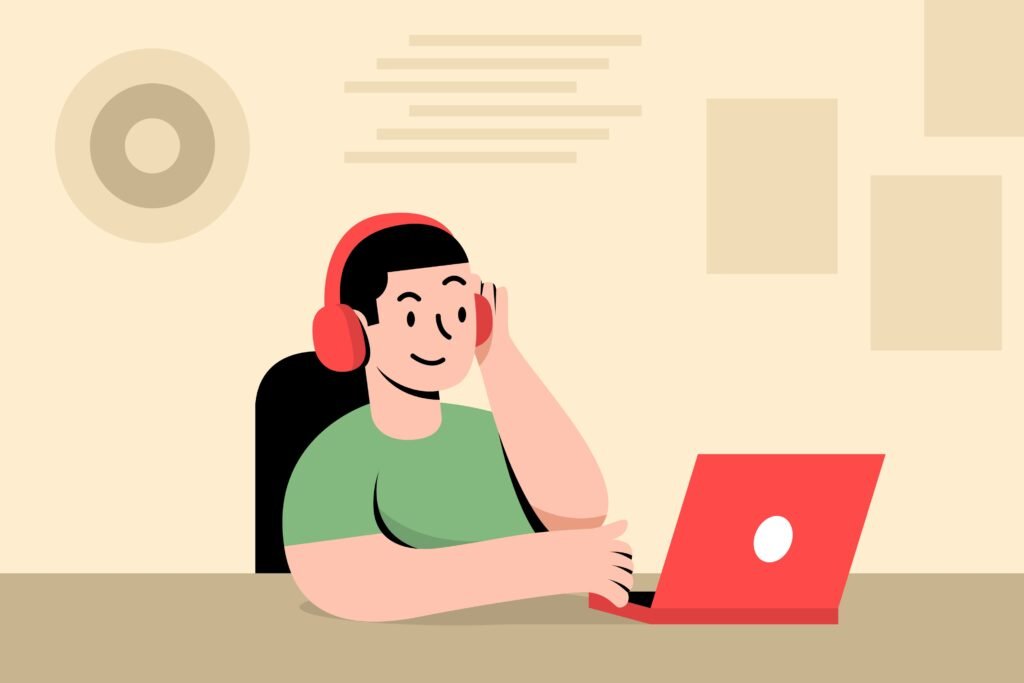
Multimodal AI, the integration of various data modalities such as text, audio, images, and video, is set to revolutionize the educational landscape. By mimicking human capabilities to process diverse sensory information, multimodal AI can enhance learning experiences, streamline administrative tasks, and offer personalized education solutions. This article explores the major applications of multimodal AI in education, from student recruitment and assessment to corporate training, highlighting practical examples and current advancements.
Understanding Multimodal AI
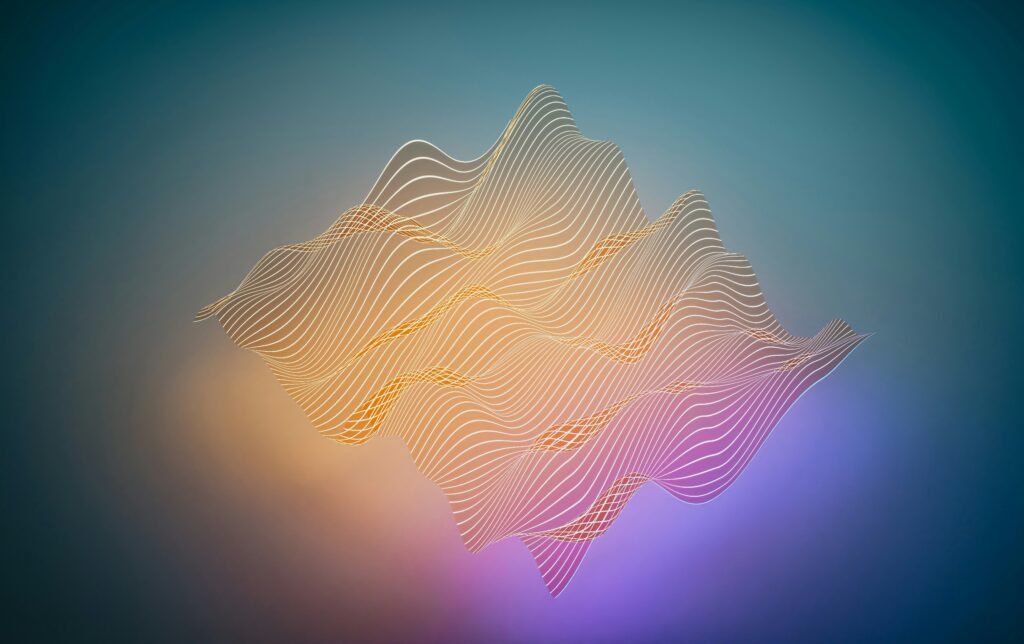
Multimodal AI is a cutting-edge technology that combines different types of information—like text, audio, images, and video—into a single system to make better decisions and provide more comprehensive insights. This is similar to how humans use their senses together to understand the world around them. By integrating various forms of data, multimodal AI can understand more complex situations and provide more accurate results, making it incredibly powerful and versatile.
Capabilities of Multimodal AI
Better Understanding Through Multiple Data Types
- Real-Life Application: Imagine a university admission process that uses not only your written application but also analyzes your video interview, social media profiles, and extracurricular activities. Multimodal AI can process all this information together to get a complete picture of an applicant.
- Why It’s Powerful: By looking at different types of data, multimodal AI can understand context better. For example, it can see if a candidate’s enthusiasm in a video interview matches their achievements on paper.
More Accurate and Reliable Results
- Real-Life Application: In online learning, if a student’s webcam detects they are losing focus, the AI can adjust the difficulty of the lesson in real-time. If it misinterprets speech due to background noise, visual cues like lip movements can correct this.
- Why It’s Powerful: Combining data types helps cross-check information, reducing mistakes. If one type of data (like audio) is unclear, another type (like video) can clarify it, making the system more reliable.
Creating Engaging and Interactive Experiences
- Real-Life Application: Virtual tutors that can understand and respond to students using voice, text, and facial expressions make learning more interactive. Students can ask questions verbally, get responses via text, and see visual explanations.
- Why It’s Powerful: This makes interacting with AI more natural and engaging, similar to human interaction. Students can learn more effectively through a mix of reading, listening, and visual aids.
Holistic Analysis and Feedback
- Real-Life Application: During a student’s presentation, the AI can analyze their speech, body language, and visual aids all at once to give comprehensive feedback.
- Why It’s Powerful: By looking at all aspects together, the AI can provide more detailed and helpful feedback, improving the student’s performance in various areas.
Personalized Learning and Adaptation
- Real-Life Application: Multimodal AI can track a student’s progress across different subjects and tailor learning materials to their needs, providing more challenging tasks if they’re excelling or additional support if they’re struggling.
- Why It’s Powerful: It ensures that each student gets a personalized learning experience, which can significantly enhance their understanding and retention of information.
Why Multimodal AI is Revolutionary
Human-Like Understanding:
- Summary: Just like how we use our eyes, ears, and other senses together to understand our environment, multimodal AI uses different types of data to get a complete picture.
- Why It Matters: This holistic approach means AI can understand and respond to complex situations more effectively, making it much more useful in educational settings.
Versatility Across Fields:
- Summary: Multimodal AI isn’t just for education. It’s also being used in healthcare, entertainment, and even autonomous vehicles.
- Why It Matters: Its ability to handle different kinds of data makes it adaptable to many tasks, driving innovation and creating new opportunities across various industries.
Ready for the Future:
- Summary: As technology advances, multimodal AI will get even better at processing larger and more diverse sets of data.
- Why It Matters: This scalability means multimodal AI will remain at the forefront of technological advancements, continuing to improve and expand its applications.
Engaging Learning Experiences:
- Summary: By combining visual, auditory, and text-based information, multimodal AI makes learning more engaging and accessible to different types of learners.
- Why It Matters: This inclusive approach ensures that all students, regardless of their learning preferences, can benefit from AI-enhanced education.
Multimodal AI is set to revolutionize education by providing more comprehensive, accurate, and engaging learning experiences. Its ability to integrate various data types allows it to better understand complex situations, provide reliable results, and create interactive learning environments. As AI technology continues to evolve, the applications of multimodal AI in education will expand, offering new opportunities for growth and innovation. This revolutionary approach promises a more personalized and effective educational landscape, benefiting educators, students, and institutions alike.
Why Multimodal AI is Important
Multimodal AI is crucial because it enhances understanding and interaction by integrating diverse data types such as text, audio, images, and video. This comprehensive approach allows AI to make more accurate decisions, provide personalized feedback, and create engaging, interactive learning environments.
Applications in Education
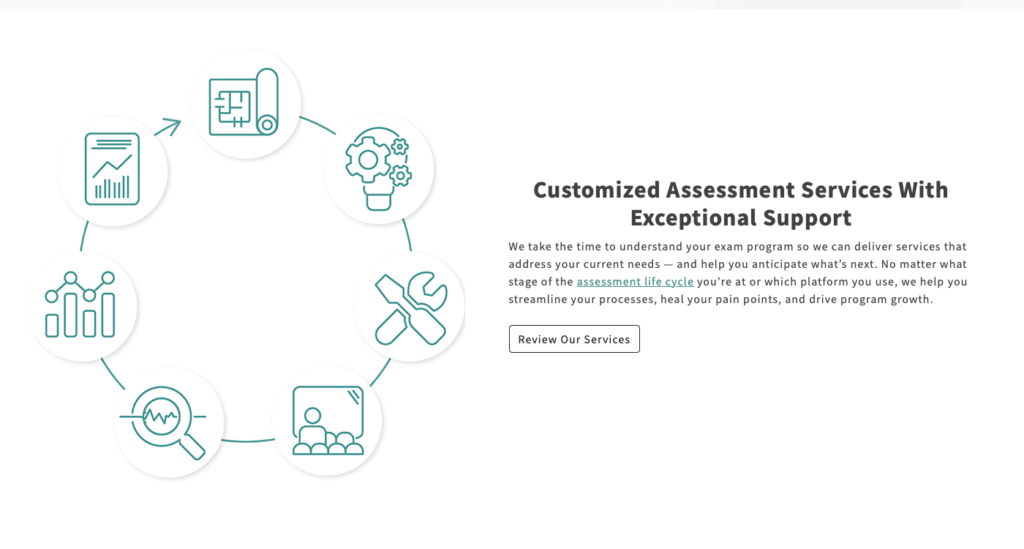
This is all great but what are some practical applications of multimodal AI in Education & how can we leverage this in a real-world scenario?
1. Student Recruitment for Universities
Enhancing Recruitment with Multimodal AI: Universities can utilize multimodal AI to streamline and enhance their recruitment processes. Traditional recruitment relies heavily on textual data from applications and standardized test scores, but multimodal AI can incorporate additional data such as video interviews, social media profiles, and extracurricular activity records.
- Video Interview Analysis: AI can analyze video interviews to assess candidates’ communication skills, emotional intelligence, and suitability for specific programs. For instance, platforms like HireVue use AI to evaluate video interviews by analyzing facial expressions, speech patterns, and body language.
- Social Media Insights: By integrating data from applicants’ social media profiles, AI can provide a more holistic view of their interests, achievements, and character, aiding in the selection process.
Impact:
- Improved Candidate Matching: Universities can better match applicants with suitable programs, improving student satisfaction and retention.
- Bias Reduction: AI-driven recruitment processes can help mitigate biases by focusing on a wide range of data points beyond traditional metrics.
2. Assessment and Proctoring
Innovative Assessment Methods: Multimodal AI can transform how assessments are conducted and monitored, providing more comprehensive evaluations of student performance.
- Automated Proctoring: AI-powered systems like ProctorU / Measure Learning use computer vision to monitor students during exams, detecting potential cheating by analyzing video, audio, and behavioral cues.
- Dynamic Assessments: AI can create adaptive assessments that change in real-time based on the student’s performance. For example, EdTech platforms can adjust the difficulty of questions during an exam to better gauge a student’s understanding and capabilities.
Impact:
- Enhanced Integrity: Automated proctoring ensures the integrity of online exams, maintaining academic standards.
- Personalized Feedback: Dynamic assessments provide immediate, tailored feedback, helping students understand their strengths and areas for improvement.
3. Corporate Training
Advanced Training Solutions: Corporate training programs can significantly benefit from multimodal AI, particularly in areas requiring nuanced skills such as negotiations and presentations.
- Simulation-Based Training: AI can create realistic simulations for training employees in negotiation and presentation skills. For instance, platforms like Mursion use AI-driven avatars to simulate real-world scenarios, allowing employees to practice and receive feedback in a safe environment.
- Performance Analytics: AI can analyze video recordings of training sessions to provide detailed feedback on body language, speech clarity, and engagement levels.
Impact:
- Effective Skill Development: Employees can develop critical soft skills through immersive, interactive training experiences.
- Continuous Improvement: Detailed performance analytics enable continuous improvement by identifying specific areas for development.
Multimodel AI Applications in Education
Student recruitment for universities: Integrates data from video interviews, social media profiles, and extracurricular activities to offer a comprehensive candidate assessment, improving matching and reducing biases.
Assessment and proctoring: Multimodal AI ensures academic integrity with automated proctoring systems that monitor exams through video, audio, and behavioral analysis.
Corporate training: Multimodal AI enhances skill development through realistic simulations and performance analytics, enabling employees to practice critical skills like negotiation and presentations in safe environments and receive detailed feedback for continuous improvement.
Models and Tools in Multimodal AI
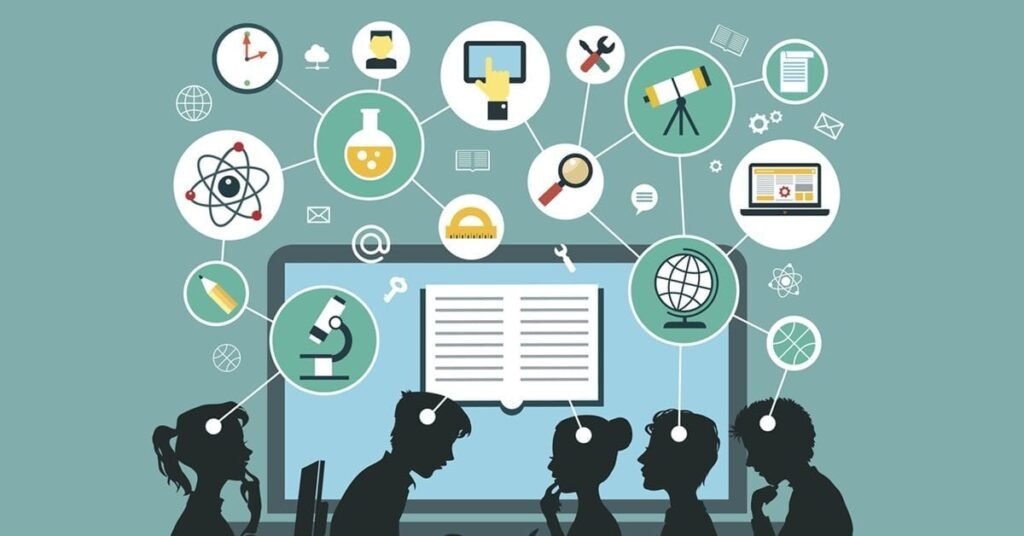
Which Models are Best for Multimodal AI?
Several advanced multimodal AI models are currently leading the way in educational applications:
Google Gemini: Known for its capability to process and integrate text, images, and audio, enhancing interactive learning experiences.
GPT-4V: An extension of GPT-4, integrating vision capabilities to understand and generate text-based on visual inputs.
Meta’s ImageBind: This model integrates six modalities—text, images/videos, audio, 3D measurements, temperature data, and motion data—learning a unified representation across these diverse data types.
Multimodal AI leverages various data types to create comprehensive and accurate AI models. Recent advancements have led to the development of several powerful models and tools designed to integrate text, images, audio, and video data. These models are pushing the boundaries of what AI can achieve in education and other fields. Here’s a look at some of the latest and most significant multimodal AI models
1. Llama 3 & 3.1
Developer: Meta AI
Release Date: April 2024
Number of Parameters: 8 billion – 405 billionOverview: Llama 3.1 is the latest iteration in Meta’s series of large language models, known for its ability to process and integrate text, images, and other data types effectively. Llama 3 models have shown superior performance in various benchmarks, including MMLU (Measuring Massive Language Understanding) and ARC (AI2 Reasoning Challenge).
Capabilities:
- Enhanced Context Understanding: Improved ability to grasp the context of conversations and provide more accurate responses.
- Multimodal Integration: Capable of integrating data from different modalities to offer comprehensive insights and interactions.
- Open Source: Available to the community for development and innovation, facilitating broader use and customization.
Applications in Education: Llama 3 can be used to create more interactive and personalized learning experiences, such as AI-driven tutoring systems that understand and respond to students using a combination of text, voice, and visual cues.
2. Gemini 1.5
Developer: Google DeepMind
Release Date: February 2024
Number of Parameters: Estimated 1.5 trillionOverview: Gemini 1.5 is Google DeepMind’s next-generation large language model, offering significant improvements over its predecessor. It features an exceptionally large context window, which allows it to process extensive data inputs, making it highly suitable for complex multimodal tasks.
Capabilities:
- Large Context Window: Capable of handling up to two million tokens, which includes a vast amount of text, video, and code data.
- Advanced Multimodal Processing: Integrates and processes multiple data types to deliver nuanced and contextually relevant outputs.
Applications in Education: Gemini 1.5 can be utilized to develop comprehensive educational platforms that integrate lecture notes, video tutorials, and interactive simulations, providing a holistic learning environment.
3. Stable LM 2
Developer: Stability AI
Release Date: January 2024
Number of Parameters: 1.6 billion and 12 billionOverview: Stable LM 2 is developed by Stability AI, known for its contributions to AI-driven content creation. This model series includes versions with 1.6 billion and 12 billion parameters, optimized for efficiency and performance.
Capabilities:
- High Efficiency: Outperforms larger models on key benchmarks despite having fewer parameters.
- Multimodal Capabilities: Designed to handle text, image, and video data, enabling it to generate rich, multimodal content.
Applications in Education: Stable LM 2 can be used to create interactive educational materials, such as video lessons and simulations, which adapt to students’ learning styles and progress.
4. Mixtral 8x22B
Developer: Mistral AI
Release Date: April 2024
Number of Parameters: 141 billion (39 billion active)Overview: Mixtral 8x22B is Mistral AI’s latest large language model, employing a sparse mixture-of-experts (SMoE) architecture. This model uses only a portion of its parameters at any given time, optimizing performance while maintaining high capacity.
Capabilities:
- Efficient Multimodal Processing: Uses active parameters efficiently to process and integrate multiple data types.
- High Scalability: Designed for large-scale applications, making it suitable for complex and data-intensive tasks.
Applications in Education: Mixtral 8x22B can support large educational institutions by managing extensive data from various sources, such as student records, learning materials, and performance analytics, to provide personalized learning experiences.
5. Inflection-2.5
Developer: Inflection AI
Release Date: March 2024
Number of Parameters: Not disclosedOverview: Inflection-2.5 powers Inflection AI’s conversational assistant, Pi, known for its conversational abilities and efficiency. This model achieves high performance with relatively fewer training resources.
Capabilities:
- Efficient Resource Use: High performance with optimized use of training data and computational resources.
- Multimodal Communication: Supports seamless integration of text, speech, and visual data for more natural interactions.
Applications in Education: Inflection-2.5 can be integrated into educational platforms to provide real-time assistance to students, helping them with queries and interactive learning modules.
6. LLaVA
Developer: Microsoft Research and University of Wisconsin-Madison
Overview: LLaVA combines visual and textual data to enhance educational tools. This model is particularly effective in creating immersive learning experiences by integrating visual aids with textual explanations.
Capabilities:
- Visual-Text Integration: Processes and combines visual data with text for enriched learning content.
- Interactive Learning: Facilitates the creation of interactive educational tools, such as virtual labs and visual storytelling.
Applications in Education: LLaVA can be used to develop virtual science labs and interactive history lessons, where visual data enhances textual content, making learning more engaging and effective.
The Future of Multimodal AI in Education
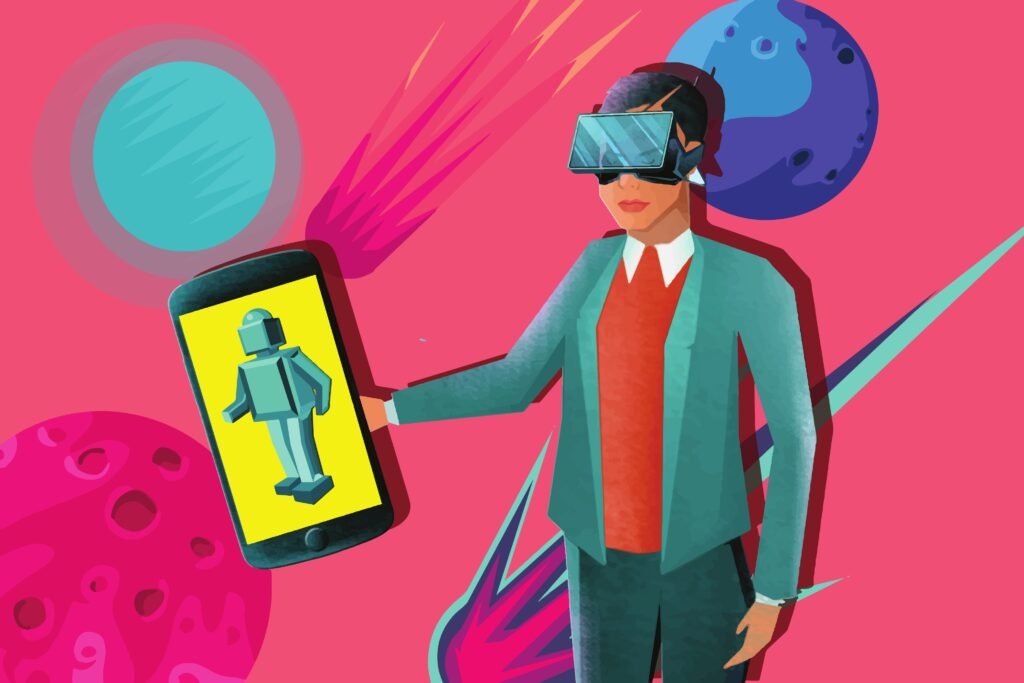
As AI research advances, multimodal AI is expected to transform education even more profoundly, leading to several potential developments that will redefine how learning and teaching occur.
Integration with IoT
The rise of the Internet of Things (IoT) will generate numerous data streams for multimodal AI systems to analyze, leading to richer insights and more personalized educational experiences. For instance, classrooms equipped with IoT devices can collect real-time data on student engagement, environmental conditions, and learning activities. Multimodal AI can process this data to adjust the learning environment dynamically, such as optimizing lighting and temperature for concentration or identifying when a student needs additional support. This integration will create a more adaptive and responsive educational setting, enhancing student outcomes and satisfaction.
Enhanced Deep Learning Models
Improved deep learning models will enable more efficient and accurate processing of multimodal data, further enhancing the capabilities of educational tools. These models will be capable of analyzing vast amounts of data from various sources—textbooks, video lectures, interactive simulations—allowing for a more comprehensive understanding of each student’s learning journey. Advanced models like Llama 406B, with its vast parameter size, will provide unprecedented depth in understanding and predicting student needs, leading to highly personalized and effective educational interventions.
Practical Applications and Future Scenarios
Interactive Virtual Classrooms: Future classrooms will be highly interactive, with AI-driven virtual environments where students can engage with 3D models, participate in simulations, and collaborate with peers in real-time. Multimodal AI will facilitate seamless interactions by integrating speech recognition, gesture tracking, and visual analysis to create immersive learning experiences.
Personalized Learning Assistants: Students will have access to personalized AI assistants that guide their learning journey, providing resources, answering questions, and offering feedback in real-time. These assistants will use multimodal data to understand the student’s context and preferences, ensuring that the support is tailored to their individual needs.
Automated Content Creation: Educators will benefit from AI tools that automate the creation of educational content, from lecture notes and quizzes to interactive simulations. Multimodal AI will generate these resources by integrating information from various sources, ensuring that content is comprehensive and aligned with learning objectives.
Real-Time Emotional and Cognitive Assessment: Multimodal AI will enable real-time assessment of students’ emotional and cognitive states by analyzing facial expressions, speech patterns, and physiological data. This capability will help educators identify students who are struggling or disengaged, allowing for timely interventions that support emotional well-being and academic success.
Challenges and Considerations
Despite its potential, the development and implementation of multimodal AI come with challenges. Integrating and synchronizing diverse data types is complex and requires robust computational power and sophisticated algorithms.
Ensuring data privacy is another significant hurdle, as educational data is sensitive and must be protected against breaches and misuse. Addressing biases in AI models is crucial to avoid perpetuating existing inequalities and to ensure that AI-driven educational tools are fair and equitable.
What does the future of education look like?
The future of multimodal AI in education is promising, with potential developments such as classroom integration, enhanced deep learning models, and broader adoption across industries set to revolutionize learning environments. These advancements will lead to interactive virtual classrooms, personalized learning assistants, automated content creation, and real-time emotional and cognitive assessments.
Conclusion
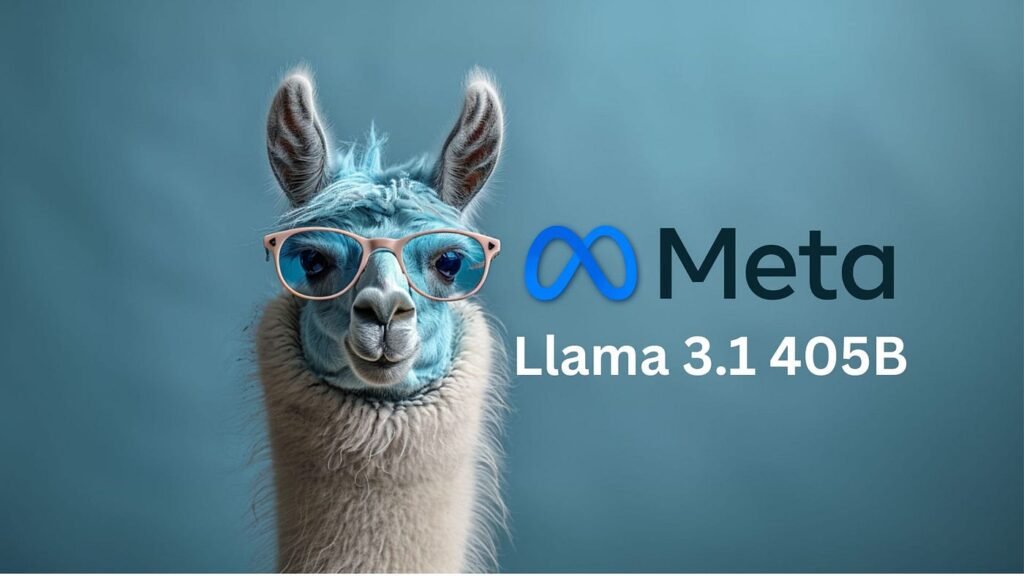
Multimodal AI is set to revolutionize education by providing more comprehensive, accurate, and engaging learning experiences. Its ability to integrate various data types allows it to better understand complex situations, provide reliable results, and create interactive learning environments. As AI technology continues to evolve, the applications of multimodal AI in education will expand, offering new opportunities for growth and innovation. This revolutionary approach promises a more personalized and effective educational landscape, benefiting educators, students, and institutions alike.
Sources: